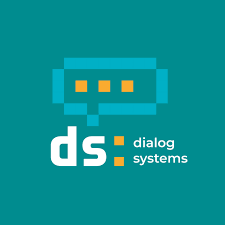
Building Dialog Systems with Less Supervision 讲座笔记
参加 Building Dialog Systems with Less Supervision 讲座笔记
- 2019 年 12 月 24 日,于华东师范大学计算机科学与技术学院,参加 Building Dialog Systems with Less Supervision 讲座
- 报告题目:Building Dialog Systems with Less Supervision
- 报告人:Zhou Yu, Assistant Professor, UC Davis
Lack of data is the number one challenge in deploying end-to-end trainable dialog systems for real-world applications. This talk will cover how to use learning methods to train a good model with less supervision. We will talk about how to integrate data augmentation, intermedia scaffolds, meta-learning to move towards the next-generation data-efficient dialog systems. We will briefly describe how to ensure the safety of the deployed system as well.
报告摘要
实际应用:与 ETS 合作;进行 PTSD 治疗
与 QA 的区别:可能存在多个来回
难点:1024 token,如何track?数据很难找到,网上的数据对我没用?domain 之间不通用?dialog 没有固定的 reference based?只能 human evaluation,真人评价?人和人不一样,难以 sample,怎么 trade off?reduce?靠增加 number of data point 还是更细致的标注?
目前已有的基本框架
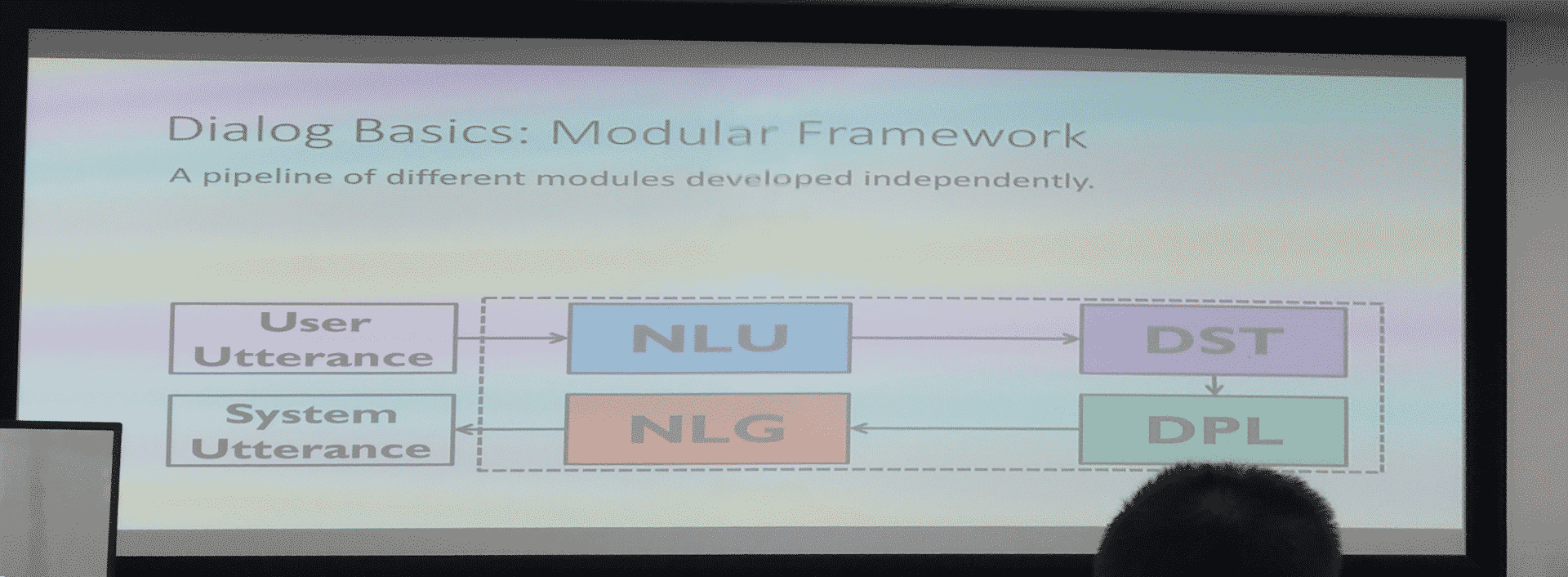
实例
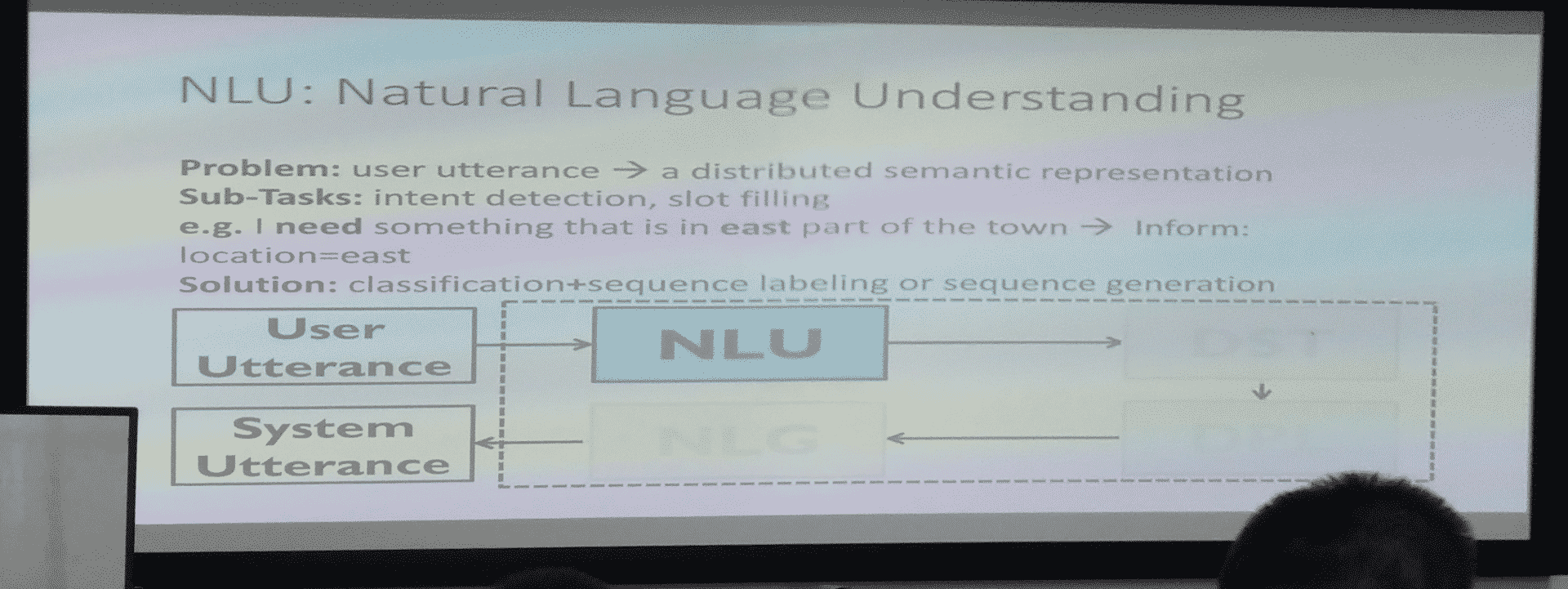
需要记住最重要的内容,例如:记住 price 要 moderate、location 是 east
知道了重要的东西以后下一步有一个计划,看到这两样东西,要知道 map 到什么地方——这一步反而简单,只要 learning 就行,最后变成自然语言,有现成的工具
存在的问题:一旦改变了任何一个环节的实现,四个 model 需要同时改变,需要 user label,即大量的人力
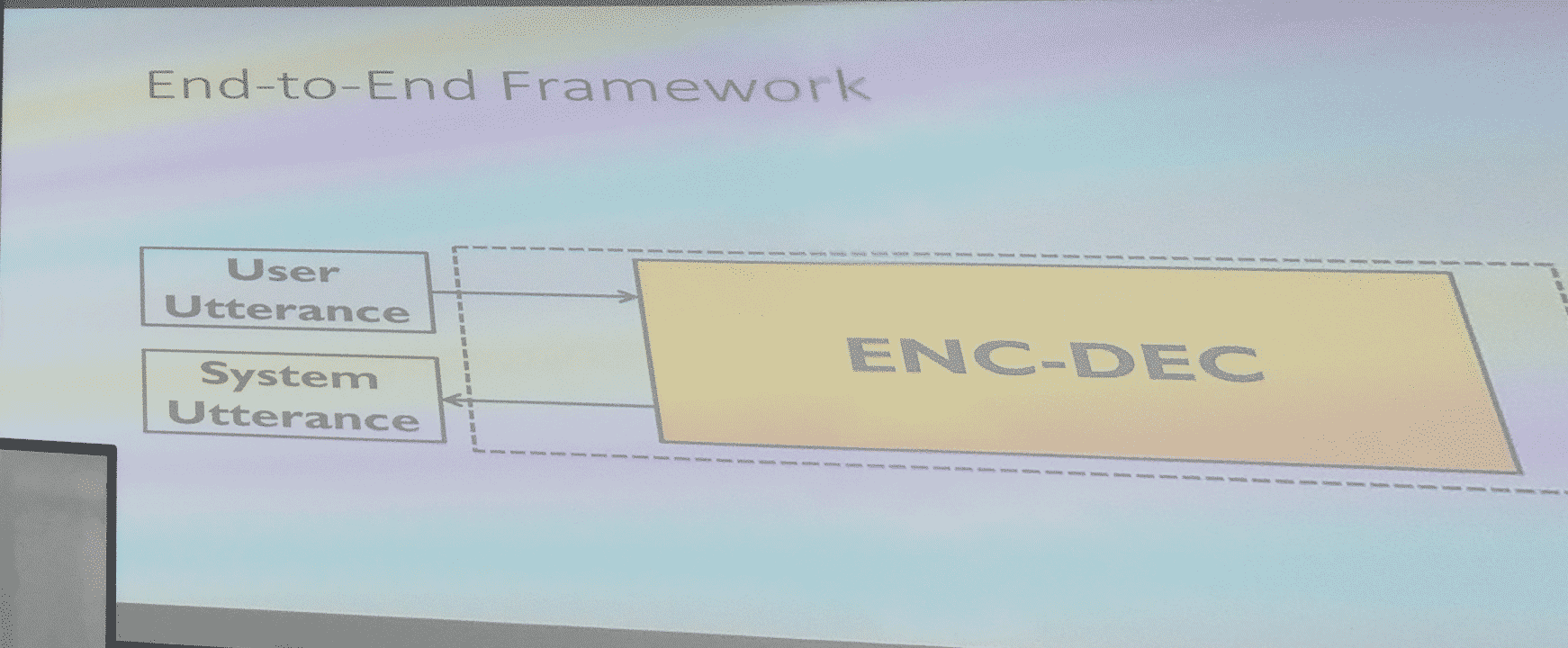
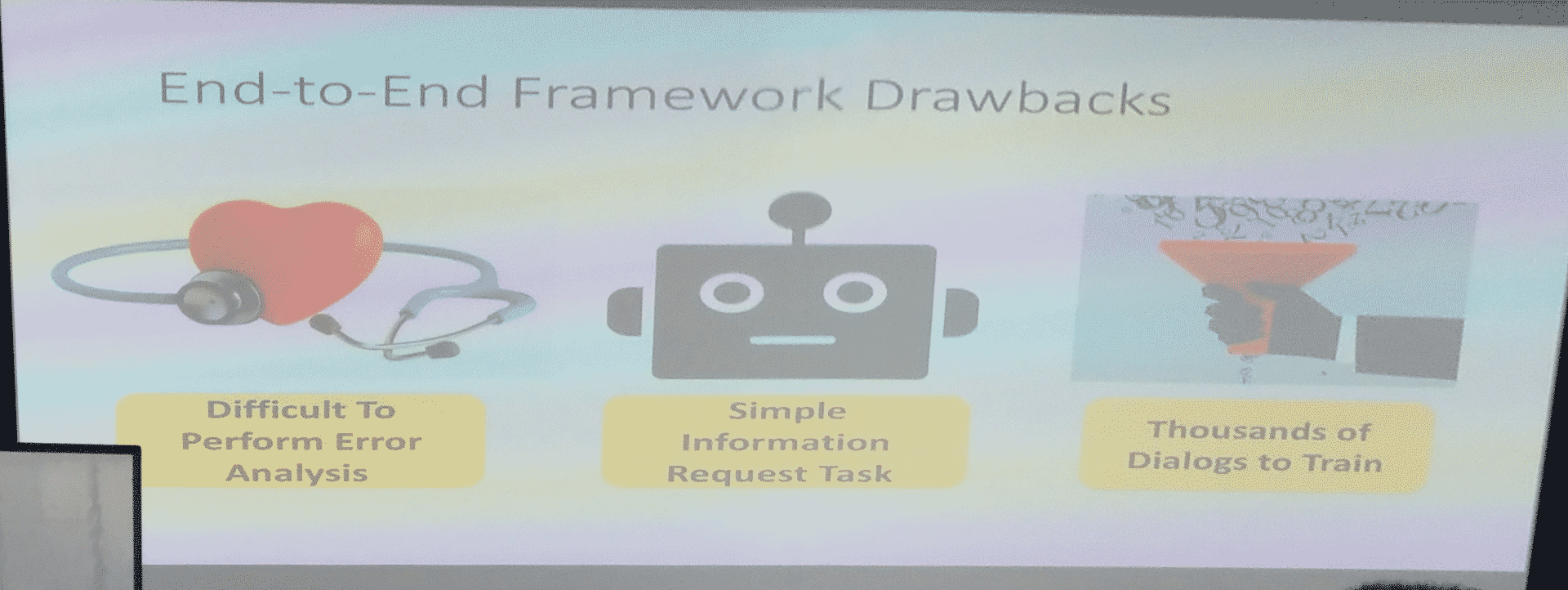
比较大的数据量,难以分析
现在俞老师的团队做的是 Plug and Play,四个 module 都可以喂,不是把 output 给下一个,而是给 state,可以 end 2 end 做 train,比较 flexible
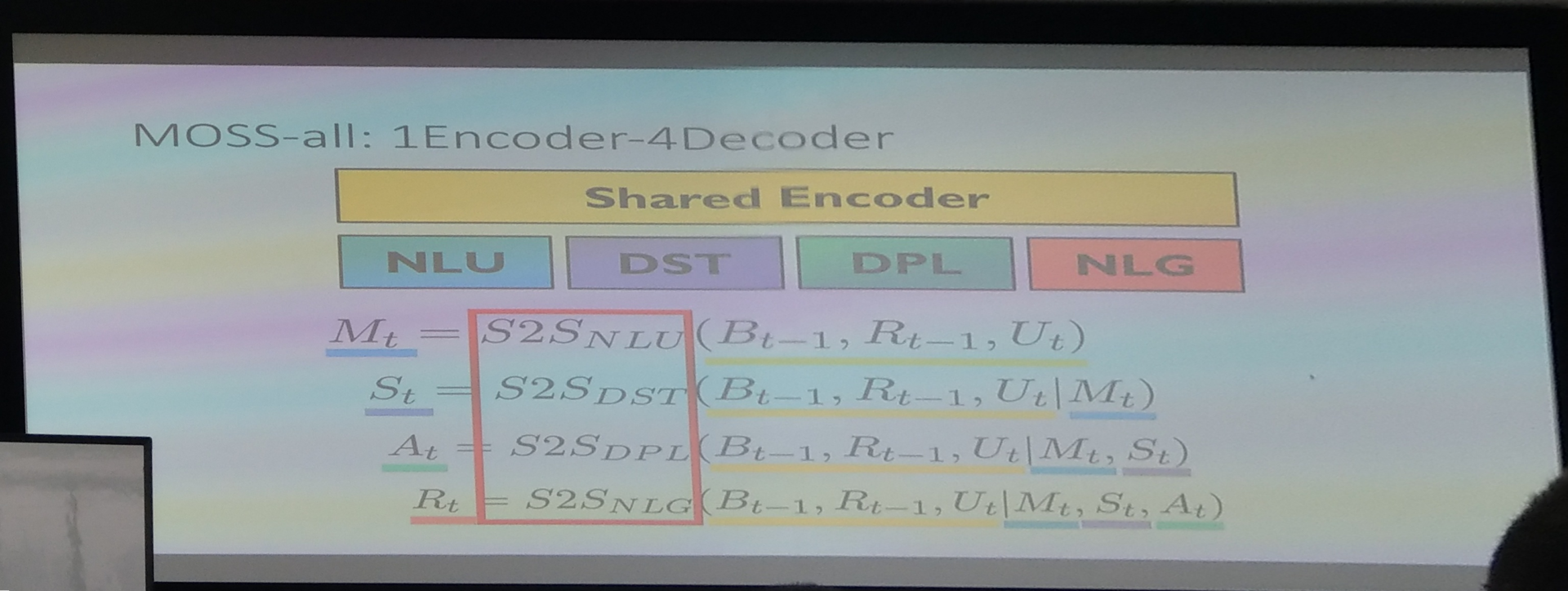
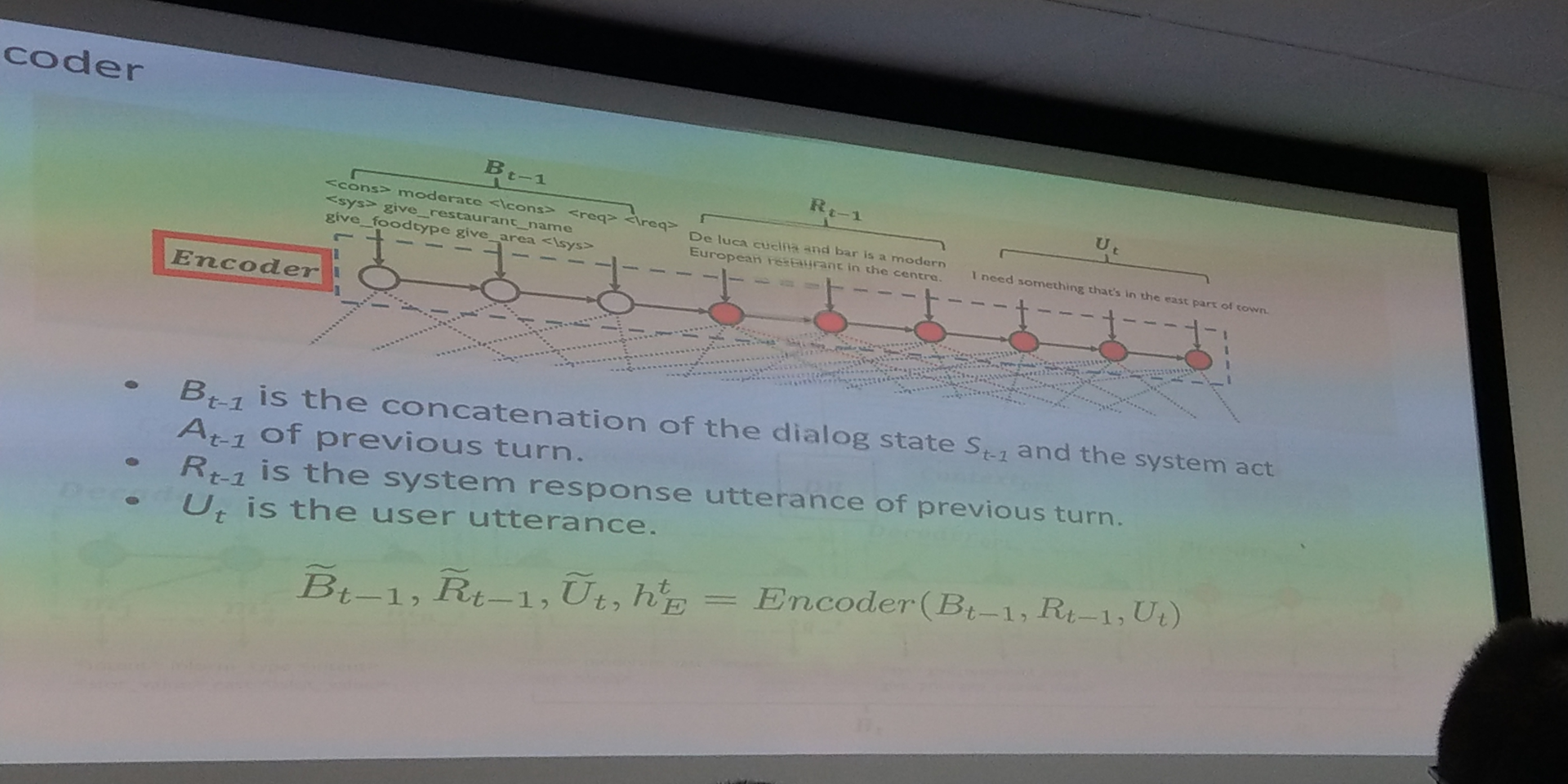
保证四个 loss 都是最小的四个,都是单独的模型,NLG 就是比较简单的,没有加其他 regular 之类的东西 60% 的数据能跑的一样好,少了 supervision
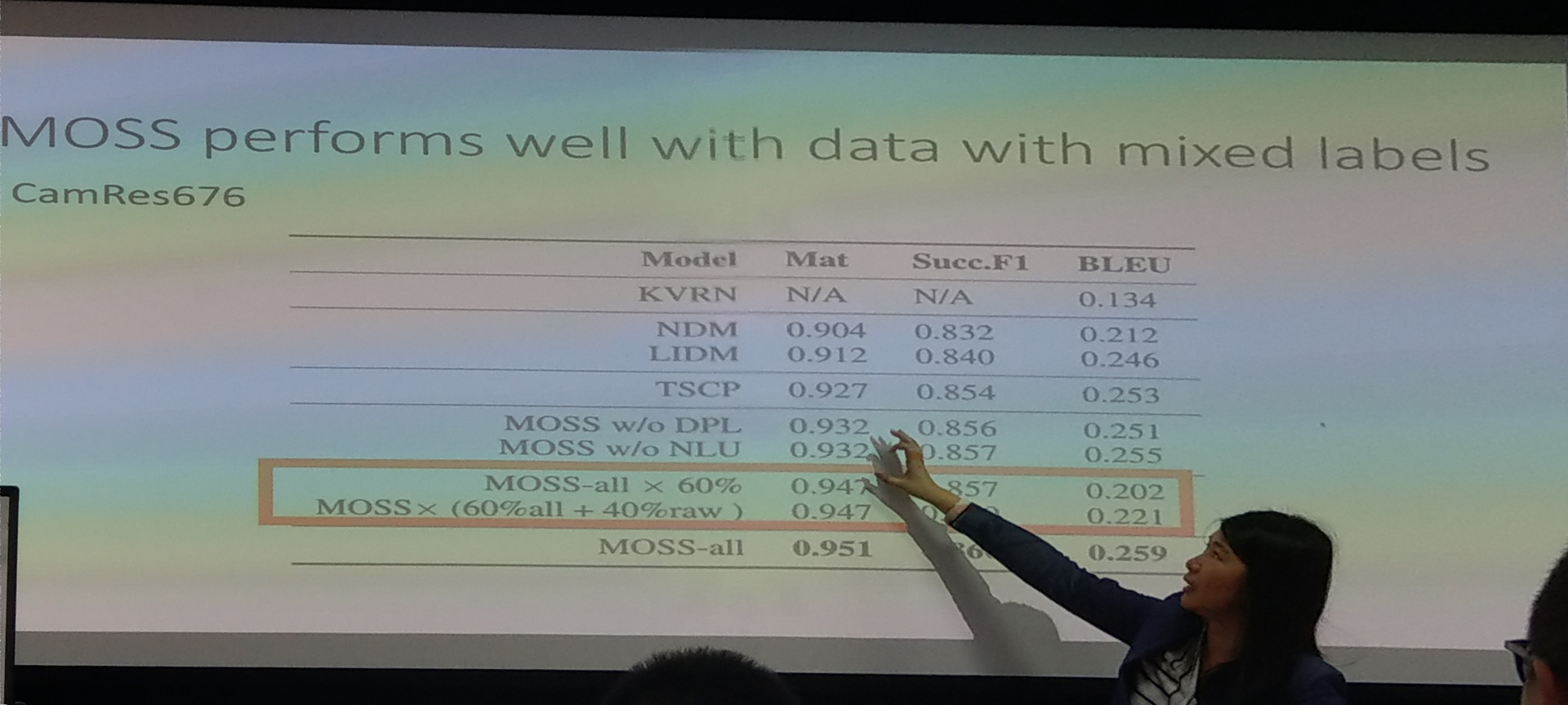
没有点数据就不 update loss
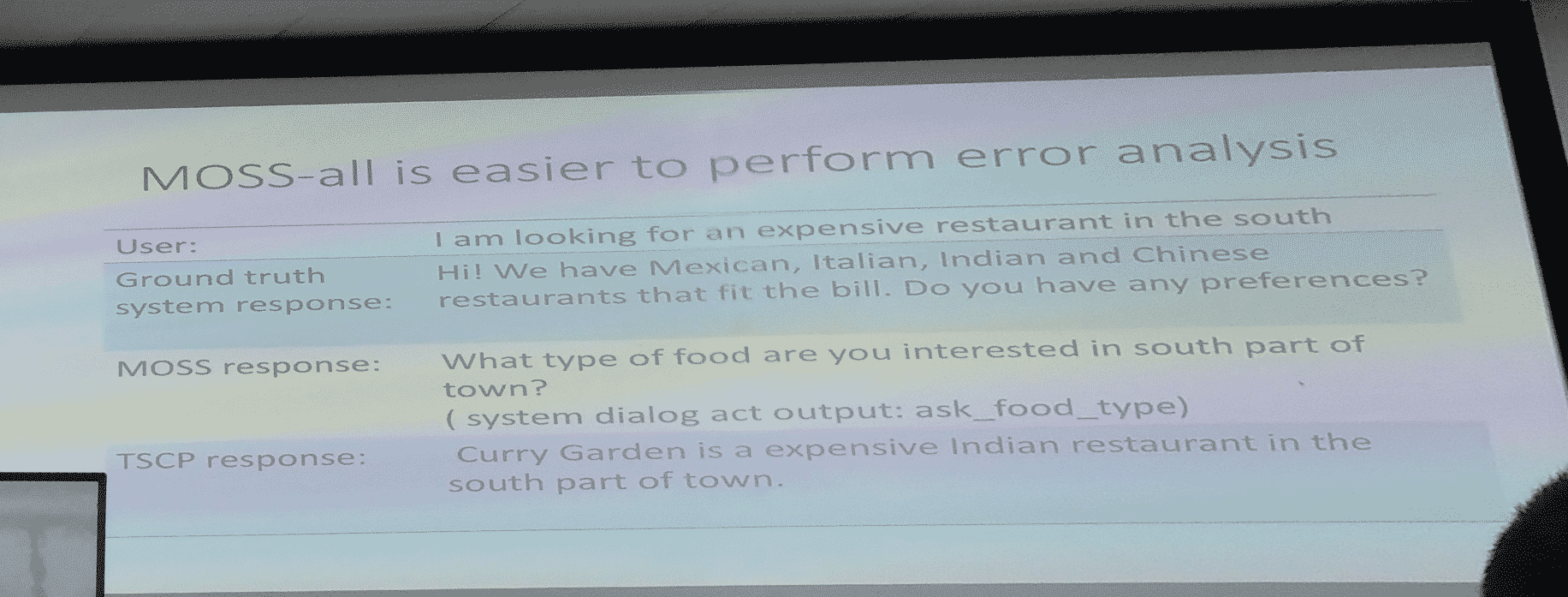
目前的优势有以下 6 点
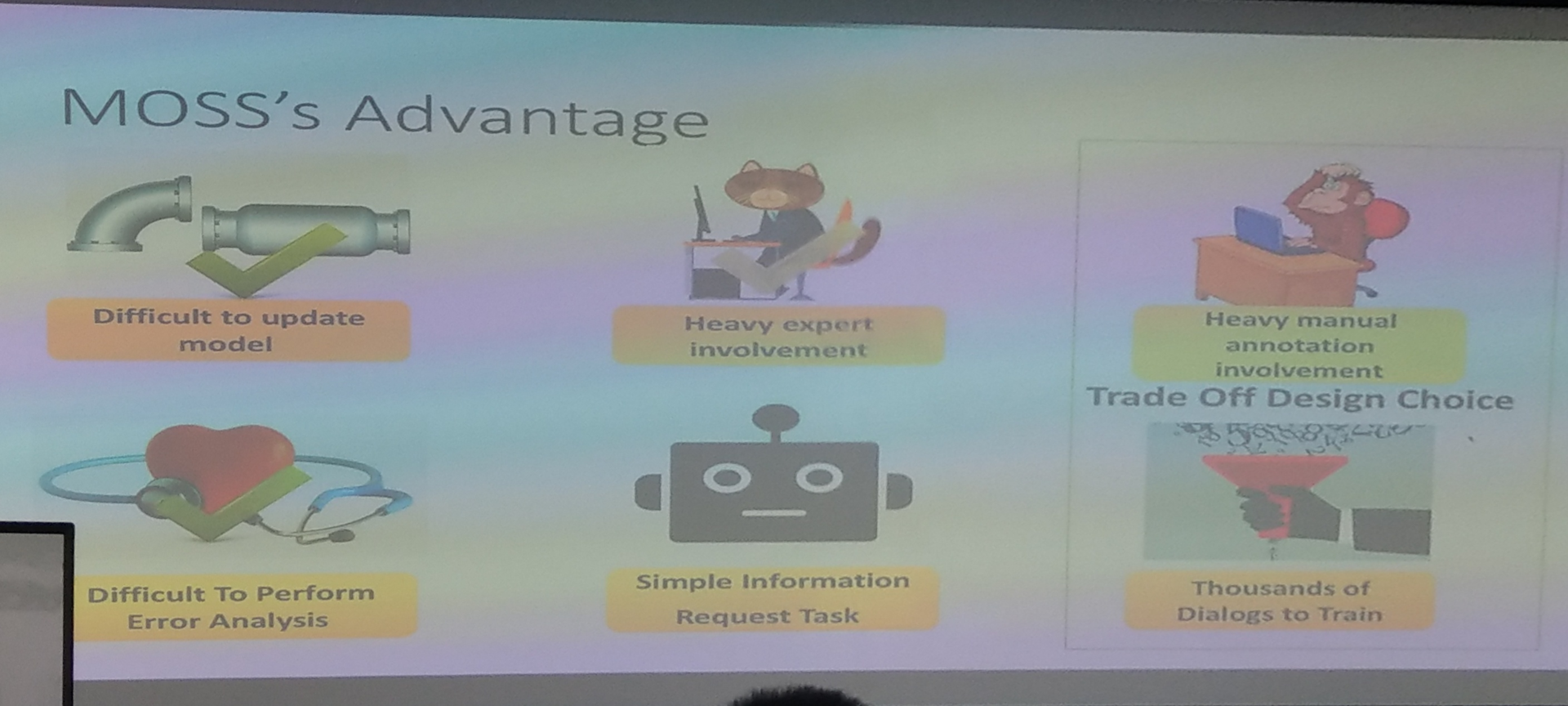
rade off 了,看需要哪些数据
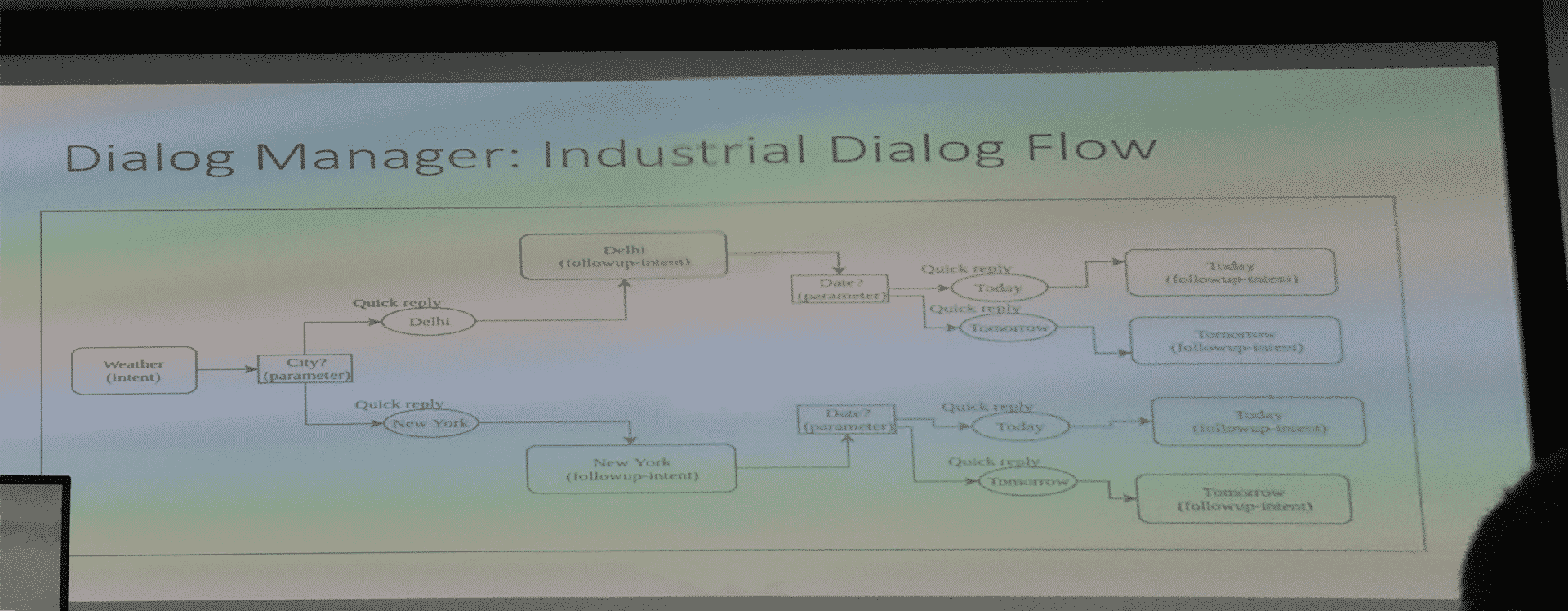
没有任何 anotation 的
用一个hmm?效果不是好,非常 sparse,viriational-rnn,变成 discrete
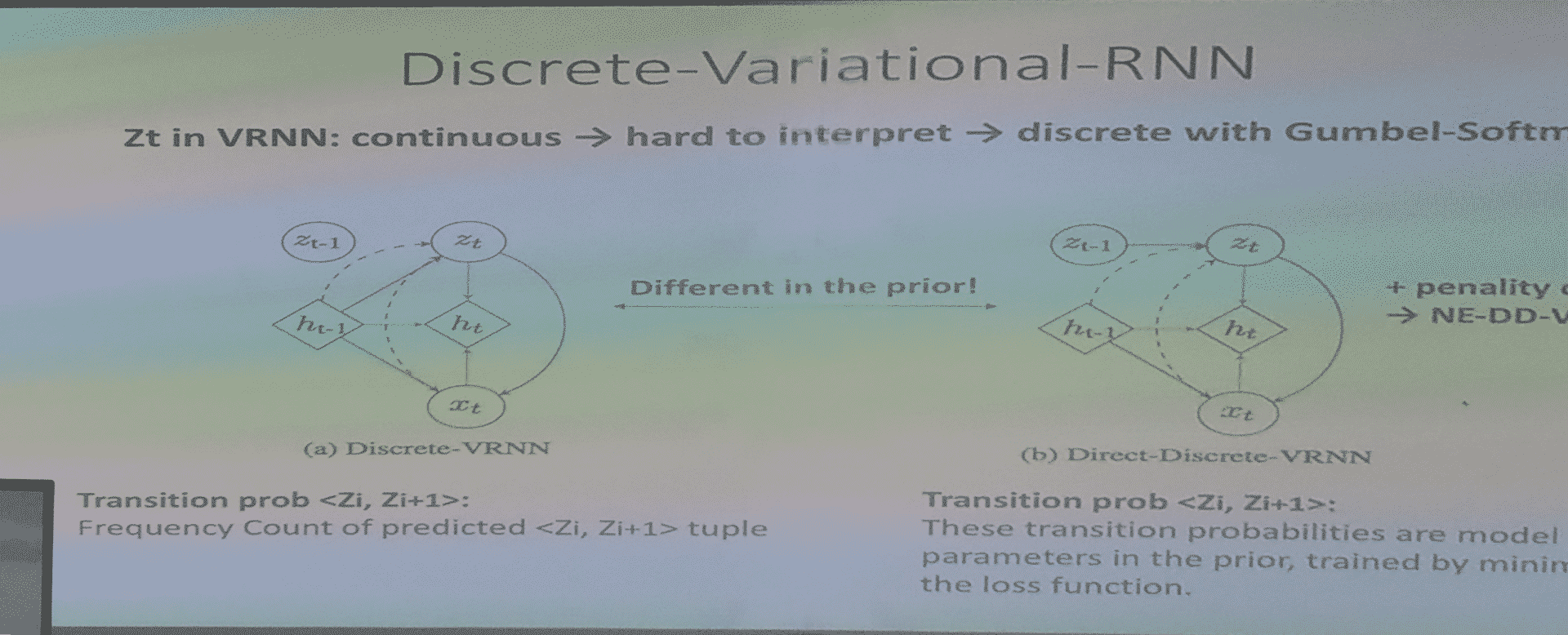
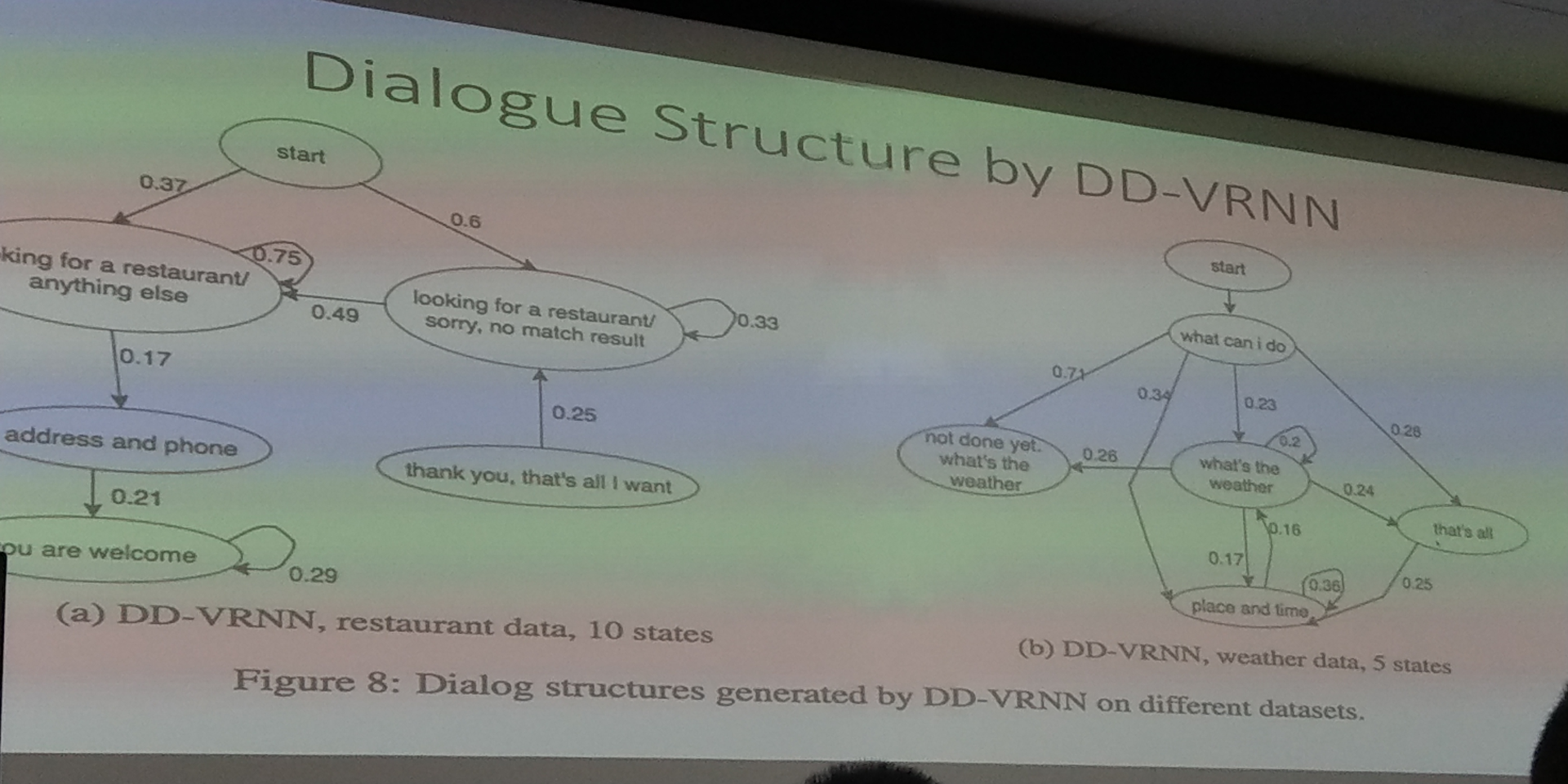
当前的处于哪一个,看 optimal 的指向哪个,divergent,之前 learning 差值做成 reward,说明还是不错的,很快就能收敛
可以和 reputation 别的 reward 合在一起、可以帮助了解没有 label 的 data
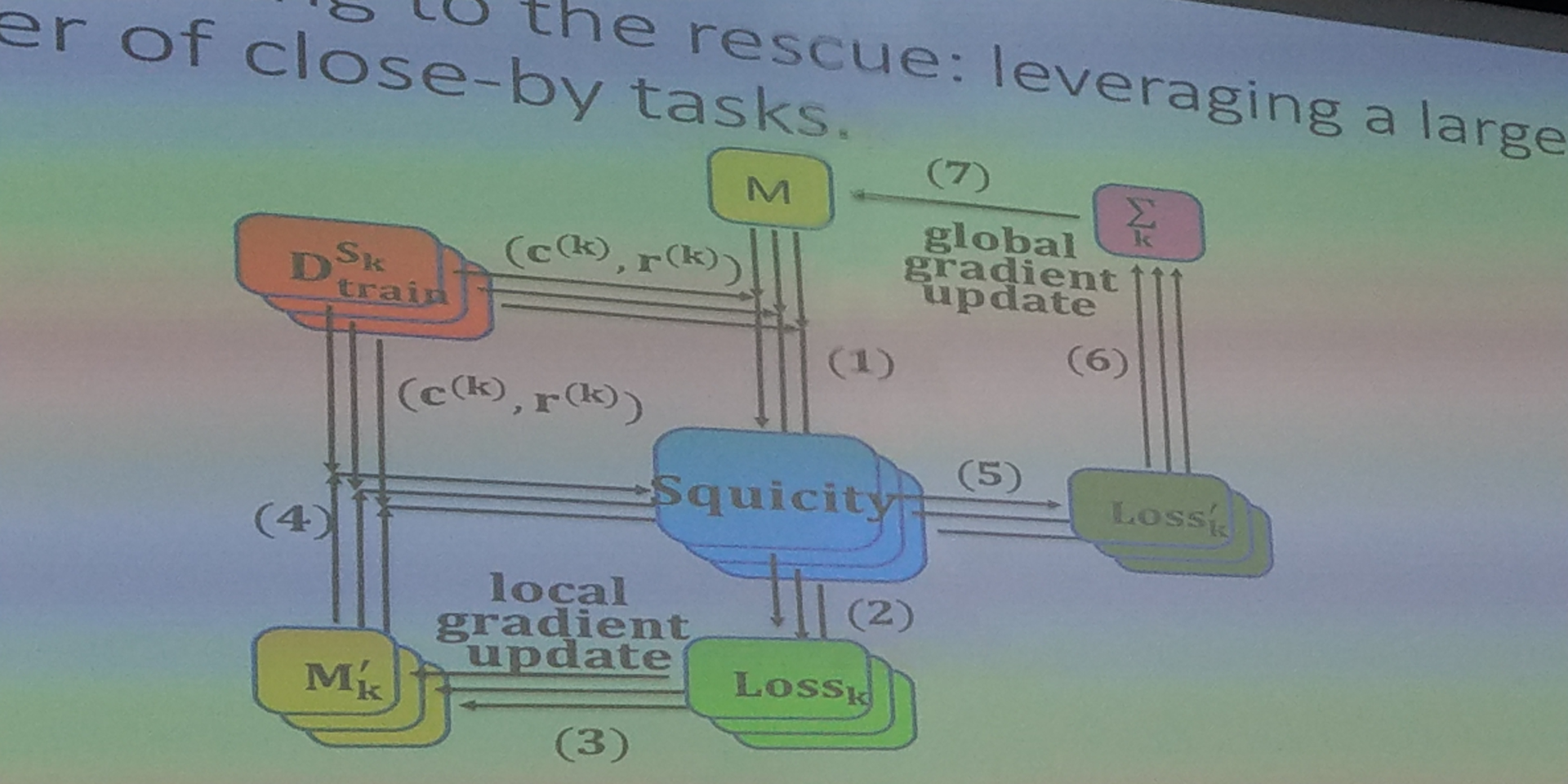
利用数据多的 domain 去提高数据少的 domain
2 step update,拉平,避免对最近做的 domain 过于多的信赖